Stepwise Landcover Classification
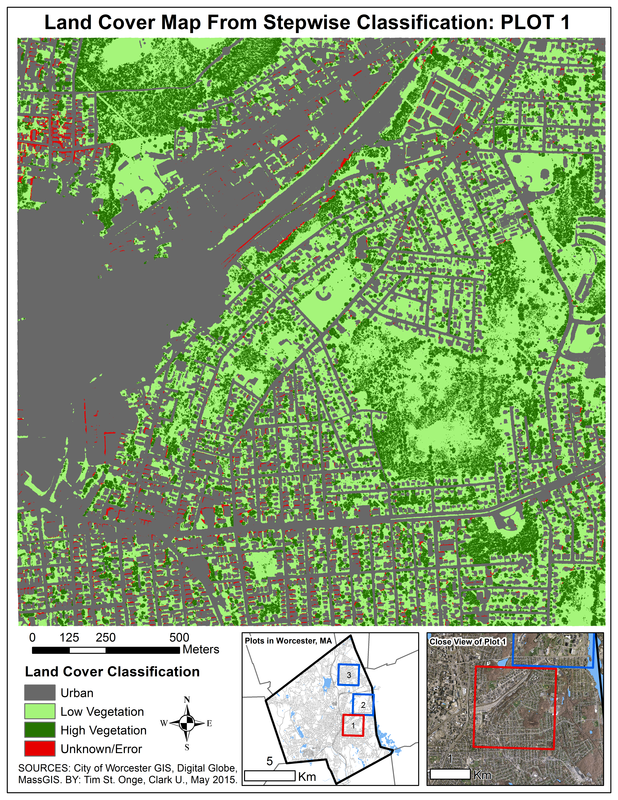
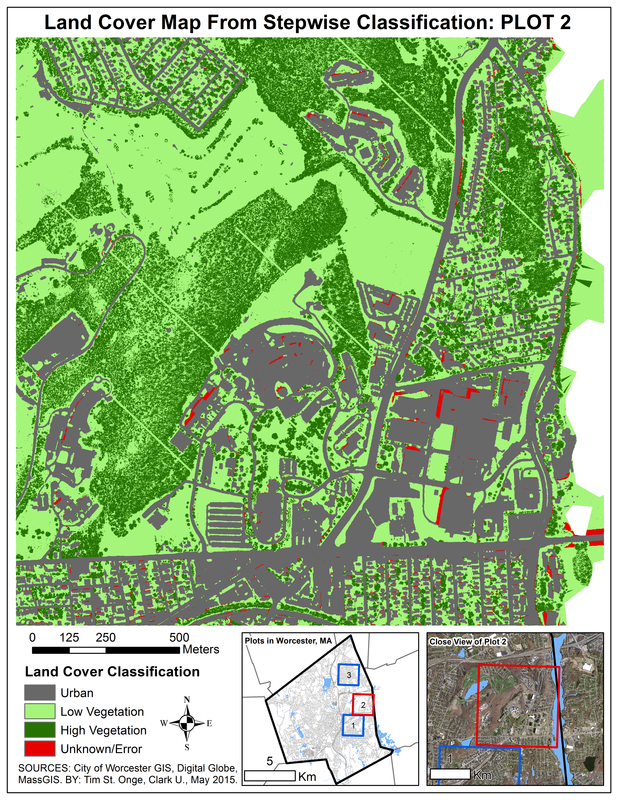
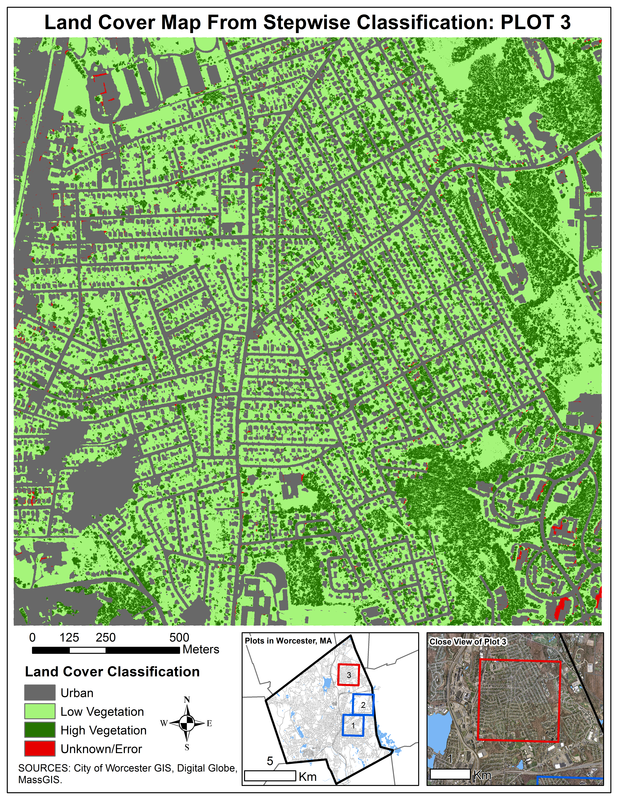
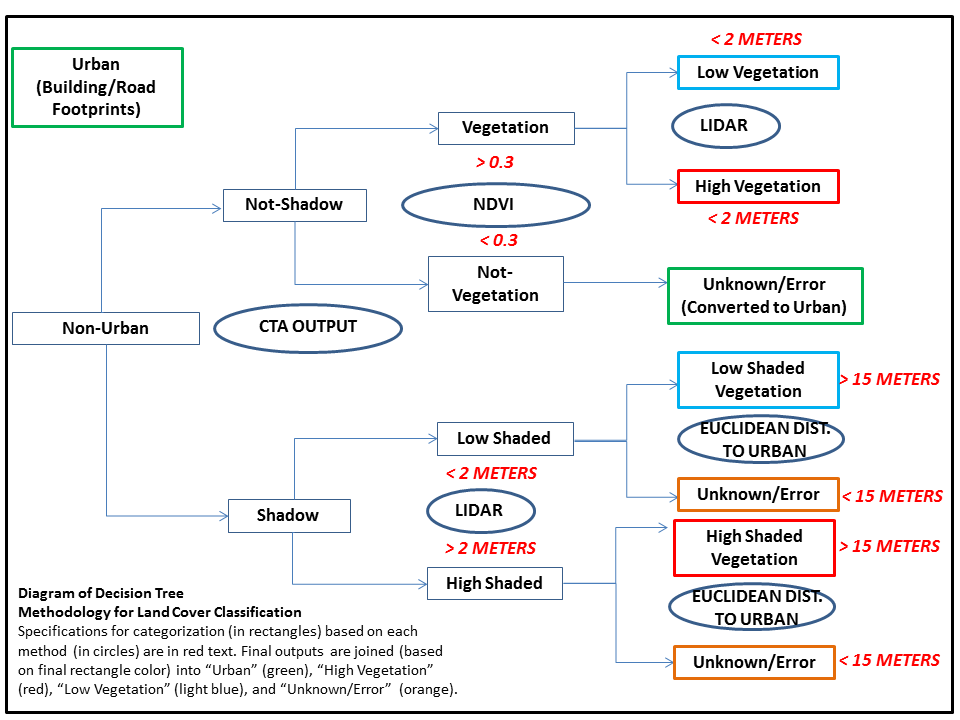
For my final research project in my Advanced Remote Sensing course, I performed a "proof of concept" study which experimented with a land cover classification scheme that relied on a decision tree structure and multiple data types to produce a highly accurate land cover map. Focusing on three locations within Worcester, Massachusetts, the study incorporated Worldview-2 imagery, LIDAR data, shapefiles, and orthoimagery. The methodology was generally based on thresholds of the Normalized Difference Vegetation Index (NDVI) to delineate vegetation, LIDAR-derived height calculations to distinguish grass and shrubbery from forest cover, and an automated Classification Tree Analysis (CTA) to specially classify areas in shadow cover in the Worldview-2 imagery. The resulting land cover maps appear to make geographic sense, and a quantitative accuracy assessment based on 3,000 sample points and comparisons to orthoimagery further supports the competence of this methodology. This study contributes to remote sensing research into stepwise classification methodologies and efforts to harness multiple earth-observing data types in the production of highly-accurate land cover maps.